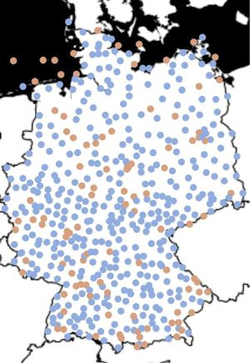
Views: 23
KIT scientists use artificial intelligence techniques to make weather forecasts more accurate
Courtesy KIT : Storms, sun, cold, heat – as the weather can be changeable, this May this year showed. Responsible is the atmosphere of the “chaotic system”: physical properties like temperature, humidity or cloudiness are constantly changing. Weather forecasts try to predict chaos and make reliable statements. Scientists of meteorology and mathematics at the Karlsruhe Institute of Technology (KIT) have developed a new method that corrects based on artificial intelligence error in the weather forecast, taking into account non-linear relationships.
Air masses that are constantly moving, temperatures that fluctuate and change the air pressure conditions make the atmosphere on us difficult to predict. But that’s what meteorologists do to make reliable weather forecasts. As a basis, they use measurements of the current state of the atmosphere and simulate alternative scenarios based on it, for example, consciously alter temperature or humidity and calculate how this modification can affect the climate. For each metric, they compare up to 50 scenarios. “Resembling the results indicated what suggests a prognosis with these relatively safe values and the state of the atmosphere in this stable and predictable area,” says Peter Knippertz of the KIT Institute of Meteorology and Climate Research.
However, this method is subject to systematic uncertainties that distort the results: “Computer scenarios do some physical relationships do not reflect the required level of detail or spatial resolution,” says Sebastian Lerch of the Stochastics Institute at KIT, which works closely collaboration with Knippertz. For example, temperature predictions in certain locations are always very light and too high for others because local conditions are sometimes time-varying and can not be supplied to models. Therefore, it would be necessary to rework the results of the simulations with complex statistical methods and specialized knowledge to obtain better forecasts and probabilities of occurrence of climatic events.
“We have developed an approach that provides better predictions than established standard methods,” according to Lerch, who works at both the Heidelberg Institute for Theoretical Studies (HITS) and a chair in the Tilmann Gneiting KIT group. The basis for this is neural networks, that is, computer programs that process information about the brain model. Artificial neurons are arranged in layers. This allows mathematicians to “train” the network to ideally process certain data. With each information flowing through the network in the training phase, it collects “experience”, can continually improve and, for example, accurately determine the probability of occurrence of local climatic events.
“We inserted in our network an intermediate layer in which the neurons analyze and evaluate the chaotic and non-linear interactions between the data of the meteorological stations and the physical states of the atmosphere in the simulation,” says Lerch. “So you can learn, for example, how changes affect temperature at a particular measuring station.” To train their network, mathematicians use climate data from Germany, which recorded 537 weather stations from 2007 to 2016. Input variables for the neural network included cloud cover, soil moisture, and temperature.
The predictions made by the network were compared by mathematicians with predictions of established techniques. “Our approach has made much more accurate predictions for almost all weather stations and is far less computationally expensive,” Lerch summarized. The advantages of neural networks as post-processing methods are, above all, to recognize nonlinear relationships independently and continuously, adapting their knowledge about them. In addition, they can process large amounts of data faster than previous methods and human experts. “This is the first time we have been able to show that neural networks are best suited to improving weather forecasts and gaining insight into weather processes.”
Inter-regional search for best weather forecasts
Lerch’s research is part of SFB / Transregios 165 “Waves to Weather” (W2W), whose co-spokesperson is Knippertz. Scientists from the Kit, the Ludwig-Maximilians-University (LMU) Munich, and Johannes Gutenberg University (JGU) as a National Coordinator work together here and in an interdisciplinary way to make weather forecasts accurate and reliable. Thus, W2W is currently the biggest challenge in predicting weather: identifying the limits of predictability in different situations and creating the best physical forecast possible. The German Research Foundation (DFG) has supported the SFB since 2015 and extended its funding a few weeks ago for another four years.
Details on the KIT Climate and Environmental Center: http://www.klima-umwelt.kit.edu
Leave a Reply